Breast Cancer Diagnosis Prediction with Machine Learning
An innovative new program at Michigan Technology University aims to improve both diagnosing and detection of breast cancer with machine learning. What does this mean for patients and the doctors who treat them?
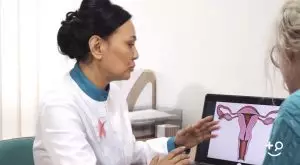
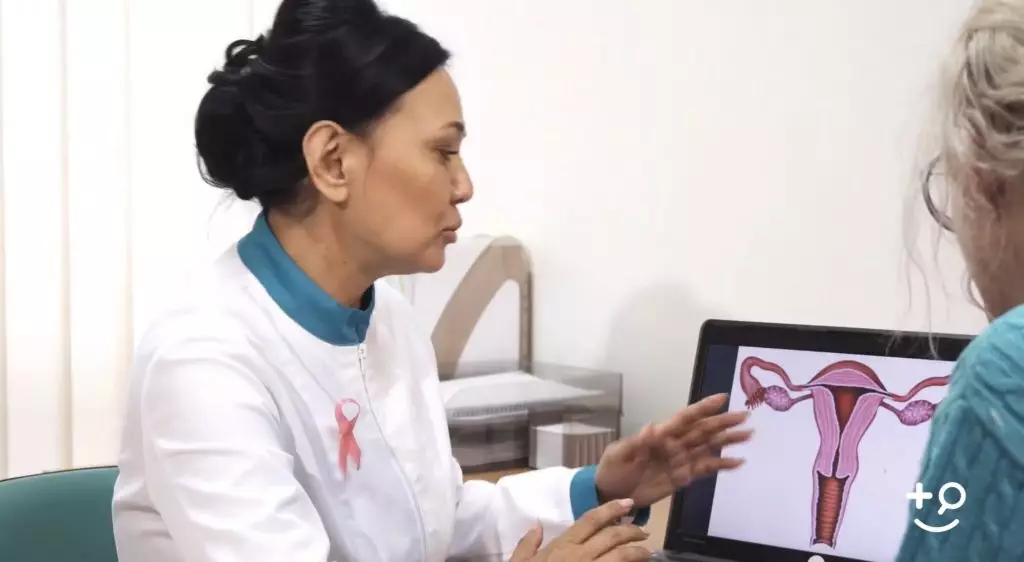
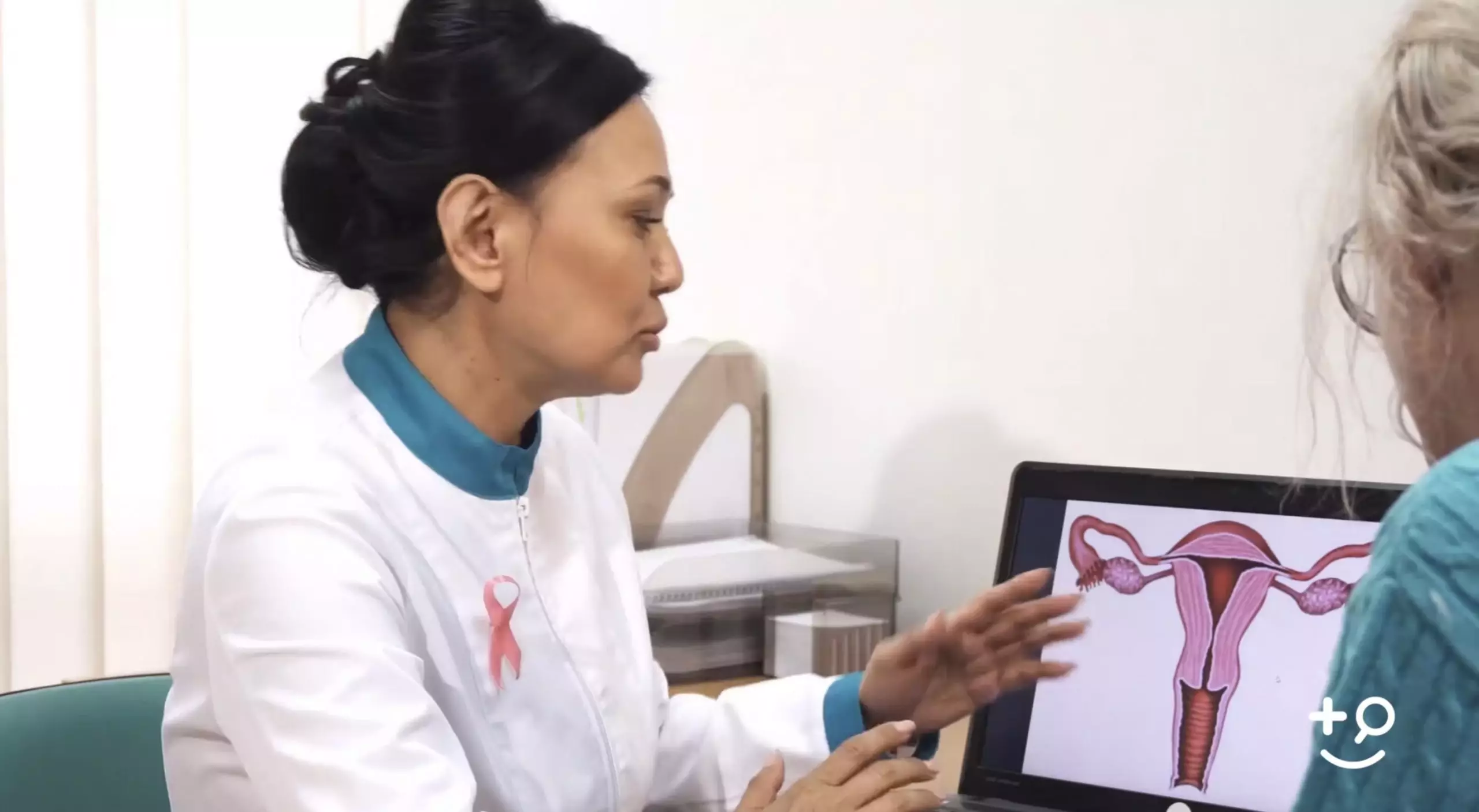
Medically reviewed by Susan Kerrigan, MD and Marianne Madsen on February 11, 2023
There’s a terrible tension for doctors diagnosing breast cancer. On the one hand, their patients could be afflicted with a very common cancer –– one with a fearsomely high mortality rate that has been reduced by early screening. On the other hand are the risks of false positives that are not only time consuming, expensive, and stressful for the patient but could, over time, discourage people from screenings. Now an innovative new program at Michigan Technology University aims to improve both diagnosing and detection of breast cancer with machine learning. What does this mean for patients and the doctors who treat them?
Breast Cancer and Early Screening
Cancer occurs when damaged cells continue to divide rather than dying off. These damaged cells can spread, attacking and killing healthy cells.This is cancer. However not every collection of damaged cells is cancerous. Some tumors are benign. Others are indolent –– so slow in their growth that they represent less of a threat than treatment. Doctors must correctly diagnose the condition or the risks can be truly life threatening.
In the U.S., other than lung cancer, breast cancer has the highest death rate and is the most commonly diagnosed cancer among women. Almost one-third of all women diagnosed with cancer are diagnosed with breast cancer, with 13% of all women expected to develop invasive breast cancer at some point in their lives. In 2021, nearly 300,000 cases of invasive breast cancer were newly diagnosed along with almost 50,000 of the non-invasive form. Over 2,500 men each year are also diagnosed with the disease. In the U.S. alone, over 43,000 women die of breast cancer. Across the world, lung cancer incidence has been surpassed by female breast cancer with over two million new cases every year.
Unlike many other cancers, like lung and pancreatic, there are ways to detect breast cancer early. Indeed, even as more cases are diagnosed, death rates have been declining with much of the reduction in mortality coming not from innovative treatments but rather from early detection. According to the Centers for Disease Control and Prevention, every two year mammograms can cut breast cancer deaths by more than one quarter for every 1,000 women screened. However, many breast cancer deaths occur in women who received a diagnosis after the period when they would have been eligible for screening.
As the American Cancer Society points out, mammograms don’t always work as screening tools. Sometimes they reveal invasive breast cancer and cancer cells in the lining of breast ducts that aren’t a true threat because they will never grow or spread. Discovering and treating these cancers –– overdiagnosis –– can be physically challenging for the patient and a terrible waste of resources for the doctor and the medical facility. Unfortunately, doctors often aren’t certain if the cancer they’ve discovered is dangerous or not. New research at Michigan Technology University hopes to change that.
Machine Learning Alters Diagnosis
As with another deadly and common cancer–prostate cancer–the issue is with both false positives and the identification of indolent or slow-growing tumors. In both cases, the patient is unnecessarily subjected to stress, expense, and even pain. Plus if a screening tool returns too many false positives people may stop using it. It’s not just about false positives. Some cancers may not pose a threat to a patient –– particularly if they are over age 65. Yet waiting has its own obvious risks since tumor growth could occur between check-ups –– especially during the COVID pandemic when many people reduced all non-emergency medical interactions.
At Michigan Technology University, researchers fed images of sampled tissue in order to teach a computer to identify patterns –– which biopsies of tumors would lead to breast cancer and which ones wouldn’t. By examining the images at the pixel level, this type of machine learning could improve not only early detection but could also motivate more women to seek early screening because of its accuracy. Researchers eventually expect that their program will not just examine tumor cells and determine whether or not they are benign but will even create a new multi-class classification of breast cancer with varied subtypes rather than just the binary benign/malignant model.
Just as with doctors, a patient’s confidence in a prediction is partly based upon the confidence of the deliverer. In other words, if the oncologist says it’s a near certainty that the cancer will spread, the patient is more likely to opt for treatment. The challenge with previous models is that there was no way to determine the computer’s confidence in its prediction. With this study, complex algorithms have been deployed to overcome the underlying weakness of machine learning-based classification models due to “the inherent randomness and the bias in the data and the scarcity of large datasets,” explains MTU’s assistant professor of mechanical engineering Susanta Ghosh. “Our work attempts to address these issues and quantifies, uses, and explains the uncertainty.”
Technology has been altering cancer treatments for some time, from robot-led surgery to complex computer imaging analysis. In the future, cancer screening could be done completely by computer. When that happens a picture really will be worth more than a thousand words.
References
- DNA damage and gene mutations
- Neoplasm (Tumor)
- U.S. Breast Cancer Statistics
- Global Cancer Statistics 2020: GLOBOCAN Estimates of Incidence and Mortality Worldwide for 36 Cancers in 185 Countries
- Cost-Effectiveness of Breast Cancer Interventions
- Limitations in the Effect of Screening on Breast Cancer Mortality
- Limitations of Mammograms
- Machine Learning Supports Breast Cancer Diagnosis Predictions